What Is Data Fabric Architecture? Simplifying Data Management Across Hybrid and Multi-Cloud Environments
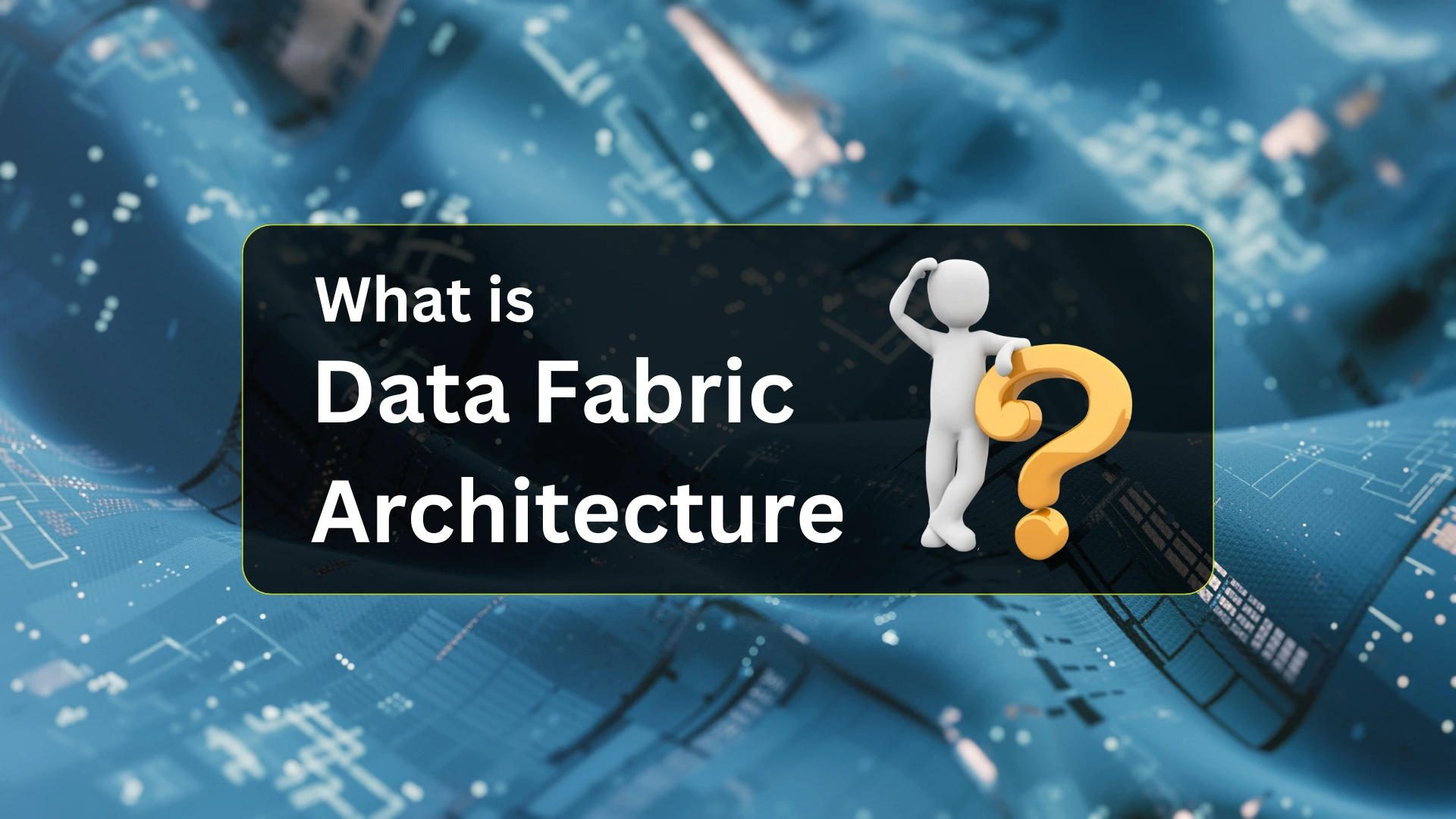
Data fabric architecture is an innovative approach to managing data in today's complex environments. It offers a unified framework that simplifies data management, allowing businesses to access, govern, and process data across various platforms—whether on-premises, in the cloud, or in hybrid systems. With data fabric, organizations can connect different data sources, break down silos, and ensure seamless data access throughout the enterprise. In an era where data is rapidly increasing in volume and complexity, data fabric architecture is becoming a popular solution that provides agility, governance, and enhanced business value. Below, we explore the concept, its significance, real-world applications, and how businesses can adopt this architecture.
Why Data Fabric Matters?
• Data Silos and Complexity: A significant challenge businesses encounter today is the rise of data silos, where information is trapped within various systems, databases, and platforms. These silos hinder comprehensive access, integration, and analysis of data. The absence of a cohesive data management strategy can obstruct decision-making and reduce operational efficiency.
Data fabric architecture tackles this issue by offering an integrated layer that links disparate data sources, regardless of their location. This comprehensive view of data enables organizations to derive actionable insights more quickly, enhancing decision-making and overall performance.
• Hybrid and Multi-Cloud Environments: As more companies embrace hybrid and multi-cloud strategies, managing data across these environments becomes increasingly complex. Each platform has its own tools, security measures, and data structures, making it difficult to achieve a unified view of the organization’s data.
Data fabric is specifically designed to operate across various environments, ensuring consistent data management, governance, and security whether the data is stored in private clouds, public clouds, or on-premises.
• Data Governance and Compliance: As regulatory requirements like GDPR and CCPA become more stringent, businesses need to implement robust data governance and privacy measures. Data fabric architecture integrates these governance principles by embedding security, compliance, and data lineage features directly into the data layer. This allows organizations to monitor data usage, track who accesses it, and identify where it is stored, aiding in compliance across various jurisdictions.
Key Components of Data Fabric Architecture
Data fabric is not merely a single technology; it encompasses a blend of various technologies, frameworks, and methodologies that collaborate to provide efficient data management. The essential components include:
• Data Integration Tools: Central to data fabric architecture are integration tools that link diverse data sources, ranging from legacy databases to contemporary cloud storage solutions. These tools consolidate data from multiple formats and systems, creating a unified view that streamlines data access and analysis.
• Metadata Management: Metadata—essentially data about data—is vital in data fabric architecture. Through effective metadata management, data fabric offers insights into the origins of the data, its structure, and its intended use. This facilitates the automation of processes such as data classification, lineage tracking, and governance.
• Data Governance and Security: Robust data governance is a fundamental aspect of data fabric architecture. By consistently applying governance policies across all data sources, the architecture ensures that only authorized personnel can access sensitive information, thus preserving data integrity and security. Automated governance also assists businesses in adhering to industry regulations.
• Artificial Intelligence (AI) and Machine Learning (ML): AI and ML are critical components in data fabric, enabling automation and intelligence. These technologies help to automate data discovery, data quality checks, and the classification of large datasets. Moreover, AI-driven insights can predict data usage patterns and optimize resource allocation, thus improving the efficiency of data operations.
Use Cases of Data Fabric Architecture
Data fabric architecture is incredibly adaptable and can be utilized across a range of industries and business functions. Here are some key use cases:
• Enhanced Data Analytics: By eliminating data silos and offering a consolidated view of all enterprise data, data fabric simplifies the process for organizations to conduct advanced data analytics. This is especially beneficial in fields like finance, healthcare, and retail, where having accurate, real-time insights is essential for making well-informed decisions.
• Customer 360-Degree View: In the realms of marketing and customer service, data fabric can integrate data from various touchpoints—such as CRM systems, social media, and customer support channels—to create a complete 360-degree view of customers. This comprehensive perspective enables businesses to tailor interactions, enhance customer experience, and boost loyalty.
•Supply Chain Optimization: Data fabric is also being leveraged to improve supply chain management. By consolidating data from different supply chain systems (like logistics, inventory, and sales data), organizations can monitor and manage their operations in real time, foresee disruptions, and make proactive adjustments.
• Healthcare Data Management: In the healthcare sector, data fabric architecture can unify patient records, treatment histories, and research data from multiple systems into one cohesive framework. This integration allows healthcare professionals to access precise and comprehensive information, enhancing patient care and facilitating advanced research initiatives like personalized medicine.
How to Get Started with Data Fabric Architecture
Implementing data fabric architecture requires a thoughtful strategy, as it involves the integration of various technologies and processes. Here are some steps organizations can take to begin:
•Assess Your Data Landscape: The initial step is to analyze the current data landscape within the organization. This involves identifying all data sources, formats, and storage systems across different environments. Gaining insight into where the data is located and how it’s utilized will aid in designing a data fabric that aligns with the organization's requirements.
• Select the Right Tools: Data fabric necessitates a blend of tools for data integration, metadata management, governance, and security. Organizations must choose technologies that can operate seamlessly across various environments and support specific objectives, such as enhanced data access, compliance, or analytics.
• Embed Governance and Security: Data governance and security should be at the heart of any data fabric implementation. By integrating governance policies into the architecture, businesses can ensure consistent data handling across the organization, minimizing risks and ensuring compliance with regulations.
• Leverage AI and Automation To fully harness the advantages of data fabric, organizations should incorporate AI and automation for tasks such as data discovery, classification, and analytics. This approach will help streamline operations and enhance the efficiency of data management processes.
Conclusion
Data fabric architecture provides a robust solution for navigating today’s increasingly complex data landscapes. By offering a unified and integrated approach to data management, data fabric allows businesses to eliminate silos, optimize operations, and make better-informed decisions. With its scalability, governance, and adaptability, data fabric is becoming a vital element for organizations aiming to future-proof their data strategies.