The Rising Tide of Optimization: How Generative Adversarial Networks (GANs) are Reshaping B2B Operations
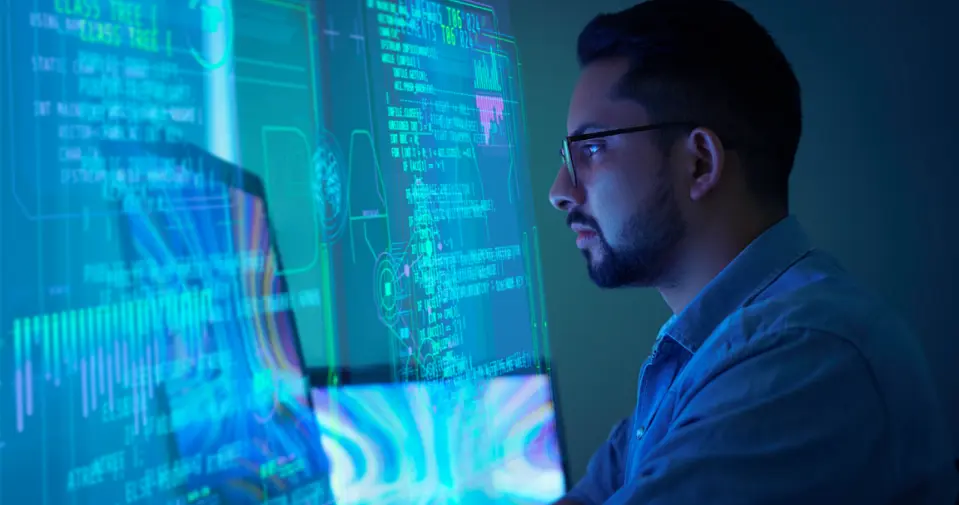
The quest for operational excellence in the B2B world has never been more pressing. Businesses face an ever-increasing landscape of complexity, requiring them to constantly adapt and optimize their processes for maximum efficiency and accuracy. In this pursuit, a new wave of technological innovation is emerging, one that harnesses the power of artificial intelligence (AI) to unlock unprecedented capabilities: generative adversarial networks (GANs).
GANs are a unique type of AI framework that utilizes a competitive training paradigm. Two neural networks, a generator and a discriminator, are pitted against each other. The generator aims to create realistic data resembling a target dataset, while the discriminator strives to distinguish between real and generated data. This continuous learning process leads to the generator producing increasingly sophisticated and realistic outputs, offering a powerful tool for B2B operational optimization in several key ways:
1. Synthetic Data Generation:
B2B operations often require vast amounts of data for training traditional machine learning algorithms. However, real-world data acquisition can be expensive, time-consuming, or even ethically questionable. GANs address this challenge by generating synthetic data that statistically mirrors real-world data. This allows organizations to train models without the limitations of real-world data constraints, enabling them to:
• Develop and test new algorithms: By creating synthetic data representing various scenarios, businesses can test and refine new algorithms before deploying them in real-world situations, minimizing risks and ensuring optimal performance.
• Enhance simulation capabilities: GAN-generated data can be used to create highly realistic simulations of complex operational scenarios, allowing businesses to test different strategies and identify potential bottlenecks before implementation.
2. Pattern Recognition and Anomaly Detection:
Identifying patterns and anomalies within operational data is crucial for optimizing processes and anticipating potential issues. GANs excel in both aspects. They can:
• Uncover hidden patterns: By analyzing large datasets, GANs can identify subtle patterns and correlations that might escape traditional methods. This allows businesses to gain deeper insights into their operations, leading to more informed decision-making.
• Detect anomalies in real-time: GANs can be trained to recognize normal operational patterns. Any deviation from these patterns can be flagged as an anomaly, enabling businesses to proactively identify and address potential issues before they escalate into major problems.
3. Scenario Optimization and Decision-Making:
The ability to explore different scenarios and their potential outcomes plays a vital role in making informed decisions. GANs provide a powerful tool for:
• Scenario exploration:By generating data representing various potential future states, GANs allow businesses to simulate different scenarios and their associated outcomes. This enables them to compare different options, assess risks and rewards, and ultimately make more strategic decisions.
• Dynamic resource allocation:In situations requiring dynamic resource allocation, GANs can be employed to predict future demand and optimize resource allocation accordingly, ensuring efficient utilization of resources and reducing operational costs.
The Impact of GANs on B2B Operations:
The adoption of GANs in B2B operations is bringing about a transformative shift. Businesses are experiencing:
• Increased Efficiency: By leveraging synthetic data, GANs can streamline data acquisition and model training processes, leading to significant time and resource savings.
• Improved Accuracy: The ability to identify hidden patterns and anomalies in data allows businesses to make more precise predictions and decisions, leading to improved operational accuracy.
• Enhanced Adaptability: The capacity to simulate different scenarios empowers businesses to adapt to changing market conditions and unforeseen circumstances with greater agility.
Challenges and Considerations:
While GANs hold immense potential, it's crucial to acknowledge the challenges associated with their implementation.
• Data Quality: The effectiveness of GANs heavily relies on the quality of the training data. Biased or incomplete data can lead to biased or inaccurate outputs.
• Explainability: Understanding the rationale behind the decisions made by GAN models can be complex. This lack of explainability can raise concerns regarding transparency and ethical considerations.
• Computational Resources: Training GANs often demands significant computational resources, which might pose a challenge for smaller organizations.
Looking Ahead: The Future of GANs in B2B Optimization
As research and development in the field of GANs progress, we can expect to see continued advancements and wider adoption in B2B operations. Addressing the current challenges through improvements in explainability, data quality control, and computational efficiency will be crucial for unlocking the full potential of this transformative technology.
In conclusion, the rise of GANs represents a significant leap forward in the pursuit of operational excellence in the B2B landscape. By harnessing the power of synthetic data generation, pattern recognition, and scenario exploration, GANs empower businesses to optimize their operations, improve decision-making, and thrive in an increasingly complex and dynamic business environment.