B2B organizations need Deep Learning Therapy to overcome their current data challenges.
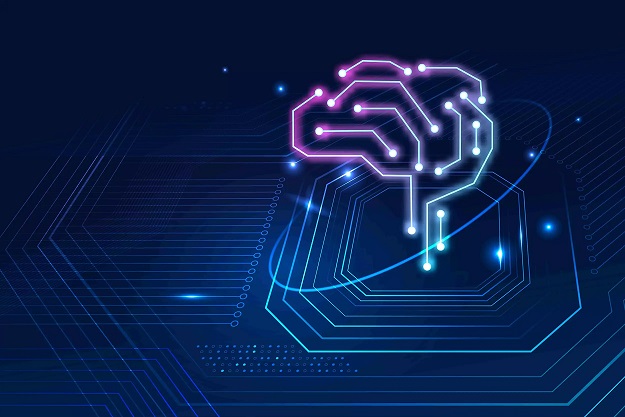
As businesses face the ongoing challenges of managing excessive amounts of data, companies are still struggling to reach a single source of truth in terms of unifying their data. Despite the great innovations in acquiring and storing data, there are deeper issues that need to be addressed to achieve this goal. B2B companies, in particular, are facing a higher order of intelligence that involves identity, relationships, and truth, which will require a more robust approach to data science.
One of the primary challenges that B2B companies face is around identity. It is essential for them to keep track of their interactions with various entities such as customers, vendors, and partners. B2B data around company deals, subscriptions, contacts, employees, and more is essential to get right. However, the traditional, offline-by-nature identities such as physical addresses, phone numbers, contact cards, and financial profiles need to be aligned with the online world, including social, web, and mobile identities.
Despite being the main conduits for B2B data, CRMs and ERPs often create multiple versions of data of the same people or companies because they rely on manual data inputs by multiple departments. There is also the challenge of a fast-growing amount of digital channels in Organizations engage with their environment through a variety of channels, such as chat, email, mobile apps, product logins, Slack channels, Zoom chats, and others. This leads to many unwanted Mickey Mouses and an exploding number of loose identities, This not only causes data inconsistencies but also hinders the ability of enterprise companies to maintain consistent engagement with their clients and partners.
The identities of B2B entities are highly variable compared to those of B2C entities, with individuals frequently switching roles and jobs and companies frequently acquiring, merging, and closing at a faster pace than before. As virtual platforms such as the Metaverse come into play, the question around ‘what is identity’ will become even more as the complexity of B2B data and identities continues to increase, resolving identity at scale will require advanced non-deterministic AI algorithms.
Another challenge that B2B companies face is understanding connections through data. Data is never singular. The B2B data is intricate, encompassing connections, history, and relationships. Like how humans are wired to connect with others, data science needs to be resilient enough to recognize and interpret these connections for added context. Identifying B2B network relationships can provide significant support for data-driven strategies.AI algorithms can be trained to detect those relationships based on implicit hints, and once machines are well trained, they can do that on an enormous scale. Although data may have limitations, the models built based on it are not necessarily constrained.
The truth complex is another challenge that B2B companies face. With a surplus of information comes an influx of misinformation, and with bots and easy access to blogs and content machines, the quality of information is decreasing. B2B companies need to train machine learning to decipher the truth. Multiple versions of one customer may leave salespeople working separately on the same account. Similar to how social media platforms employ their top data scientists to detect fake news and untrustworthy information in real-time and on a large scale, B2B organizations can use AI to detect unreliable data that may mislead their operations. AI can be highly beneficial in identifying inconsistencies and recommending the more plausible option. However, the truth may sometimes be subjective and require human supervision to guide the AI. The ideal approach would be for AI to make predictions and humans to oversee the results, and then feed the information back into the machine to enable complete automation.
The good news is that AI is constantly undergoing innovation to meet the demands of these challenges. While the data might be limited, the applications of AI are limitless. The challenges faced by businesses with their data are becoming more complex and require a combination of AI and human intervention to find solutions. The collaboration between humans and AI can help to enhance the quality of data for businesses.